How to Measure the Effectiveness of Your Data Team
What is Product Intelligence? Learn more here.
When you’re part of a data team, you hear it time and time again: the amount of data is exploding. This data holds the keys to valuable insights. Organizations that unlock these insights can create better experiences for customers and gain a huge competitive advantage.
Unfortunately, it’s not that easy for data professionals. In the early 2000s, when organizations were first going online, the novelty of a page view graph was enough to get management excited. A dashboard of clicks or “engagement” was touted as a strong insight and a staple of an analytics program.
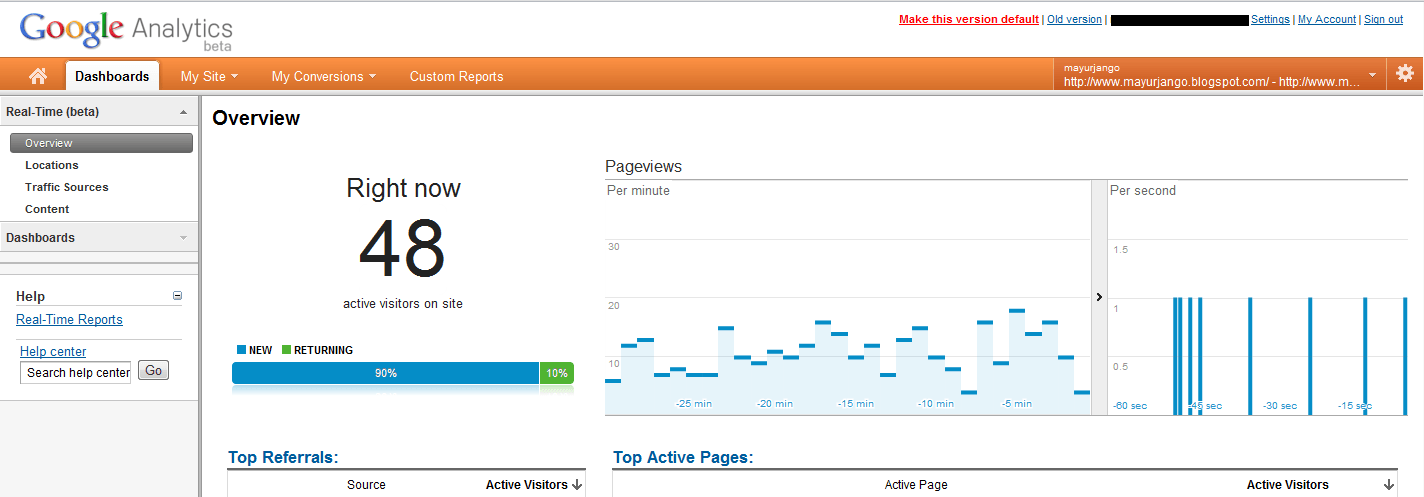
Figure 1. In the beginning of the digital world, dashboards with vague metrics like page views were often heralded as part of a true “data-driven strategy.” Now data teams are expected to deliver more — and rightfully so.
Now organizations know better and are looking for ways to use their data to drive business and true ROI. Unfortunately, they often don’t know what questions to ask of their data to get these critical insights. In an ideal world, we’d all have out-of-the-box dashboards and the same KPIs to track, but unfortunately leveraging your data is not a one-size-fits-all approach. If you try to make your metrics too basic, the value and insights won’t be there. But regardless of your industry, company size, and goals, there are still some quantitative baselines that can help you measure your team’s performance.
The results from business problems you’ve solved
Every organization is unique, but they all have challenges. Data teams should be focused on solving them. A good data team has clearly defined OKRs that align with the issues facing the business at large. For example, to support an organization’s goal to increase revenue, a data team can judge its effectiveness on whether it discovers a solution that bumps the sales win rate or increases the average order size. Similarly, if an organization struggles with customer retention, a data team can take on an OKR that improves the customer experience or increases upsell of your product.
It can sometimes be challenging to decide which KPI to align with, now that organizations often have priority lists that are 5, 10, or even 20 bullets long. What do data teams do then? It’s always a best practice to help organizations solve the problems that directly impact revenue, market share, and shareholder value. Once you’ve implemented a particular solution, you can then correlate it back to these goals.
The balance of business metrics vs. foundation building
It’s common for data teams to have a strategy that looks like this:
Step 1. Build a clean data infrastructure.
Step 2: Get great insights that improve your business.
It’s nice in theory, but if your team’s main measure of success is good architecture, you’re in trouble. You can’t set up a perfect system and sit still while the business continues on. Instead, your team should really be working on a loop philosophy — you need to build while you’re flying.
In other words, don’t put off improving business metrics in favor of optimizing your infrastructure, especially when you’re doing the small stuff. For example, when you’re deciding whether to improve your Salesforce instance operationally or help move a sales KPI like win rate or deal size, there should be an intentional balance between the two (and it should swing in favor of the business). Similarly, a newly hired data scientist or analyst should be able to find low-hanging-fruit optimizations to the business within a few weeks (if in a small or medium sized company). At the very least, hold yourself to a monthly cadence of trying to improve a business metric and force yourself to achieve a measurable improvement. Building a nice infrastructure isn’t enough. If all you’re doing is foundation work, you’ll never get out of foundation land.
How often your company follows your recommendations
Knowing a solution is only half the battle. Convincing people to use resources to implement your solution is another. As a data team, you are at the mercy of others to drive value to your organization. You can have the smartest, most innovative people on your team, and if they can’t convince others that their solutions will help the organization, they’re not effective.
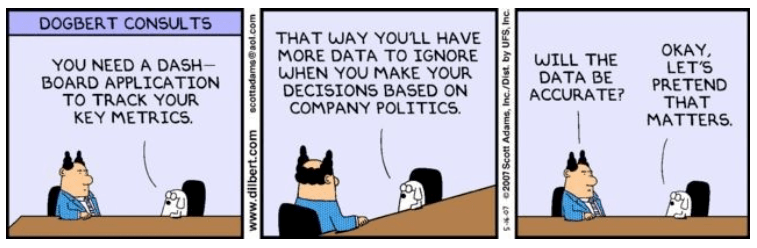
Figure 2. Effective data teams don’t just recommend solutions, they must work cross-functionally to ensure these positive changes are implemented. Otherwise even the best data insights can become lost in a world of inertia, bureaucracy, and ego.
This can sometimes be an uphill battle. Data teams are charged with finding broken things and fixing them — and it’s easy for this to appear antagonistic, especially to those who are used to systems working in a particular way.
Additionally, some data teams may be dealing with a conflicted cultural attitude toward data — some leaders want to keep their head in the sand and resist the insights gleaned from quantitative sources. But at the end of the day, you can’t argue with results. In these challenging situations, find some insights that can move the needle without much lift from other teams. When you find business problems and solve them, others will like you and respect your recommendations. Remember: most businesses succeed despite themselves. It should be relatively easy to find problems, then parse through the data and make a difference.
The rate your data team has grown
If you’re part of a data team in a fast growing company, you’re likely not going to get laid off even if you’re not producing value. But if you’re the first data hire, and you think you need three to four more people, you’ll need to justify your value. If the company finds that your team is driving value to the business, it will continue to invest in resources to support you. Therefore, team growth rate is a key indicator determine your effectiveness.
Not all data teams look alike — many companies are taking the lower-risk, lower-reward approach by embedding data professionals within different functions. This structure isn’t optimal long term, but the upside is it’s often easier to tie results into organizational metrics. If this approach is successful, as companies grow, they’ll often centralize the data resource. This evolution of the team, although not an increase in size, can also be seen as a huge investment in your team.
All in all, data teams and analytics programs have come a long way in the last few decades. But there’s still more to do prove their worth. Regardless of how you measure the effectiveness of your data team, it’s key to show business results, build relationships cross-functionally, and educate others about the potential value of data.